8 Automated driving
8.1 Automated passenger cars
Updated: 29th March 2022
Definition
The potential of automated cars is great - for society, for safety and for Europe as a business location. For society, the opportunity lies in better integrating elderly or disabled people and offering people the possibility to use the time during the journey productively or for recreation. Automated taxis or buses may run so cheaply that rural areas can also be made more accessible. Traffic runs more smoothly and goods can be transported in a more rational and environmentally friendly way. Depending on the degree of automation, the number of accidents could also be further reduced, because human error is the cause of 90 % of all accidents. However, this process will take a long time because conventional and automated vehicles will still be driving in mixed traffic for many years to come. Failures of technical systems or misjudgement of traffic situations must be avoided, henceforth, the development of the best technology for fully automated driving is immensely important. According to surveys in Germany, however, 45 % of drivers currently do not believe in the reliability of vehicle technology or are afraid of hackers (Rudschies & Kroher, 2020).
The levels of automation are currently divided into 5 levels (Paulsen, 2018):
First level: assisted driving
Individual assistance systems provide support for specific driving tasks. Assisted driving is already a reality in many cars today. (automatic adaptive cruise control and automatic lane departure warning).
Second level: partially automated driving
With semi-automated driving, the car/truck can temporarily perform some tasks itself - without any human intervention. For this purpose, various individual systems are combined with each other - in this case, the automatic adaptive cruise control with the emergency braking and lane departure warning systems. Further level 2 functions (overtaking assistant, automatic parking)
Third level: highly automated driving
Highly automated cars/trucks (Level 3) can perform certain driving tasks autonomously and without human intervention, but only for a limited period of time and under suitable conditions specified by the manufacturer. Level 3 cars will probably be on the road on motorways first: There is no oncoming traffic there, the lane markings are usually in order, and the roads are continuously recorded as digital maps. Since 2017, there has also been a legal framework for Level 3 cars in Germany: as soon as the driver puts his car into highly automated mode, he is allowed to turn his attention away from the road traffic. This means that the driver is allowed to read the newspaper.
However, if the system detects a problem and sends a signal, the driver must take over the wheel immediately.
Fourth level: fully automated driving
In the development departments of the large car companies, but also at Apple, Google or Uber, engineers and computer scientists are working flat out on the full automation of the car, i.e. level 4 on the way to autonomous driving. In this level, the technical systems carry out all driving tasks automatically, and the car can also cover longer distances without intervention.
So far, however, there is no legal framework for fully automated vehicles.
Fifth level: autonomous driving
The fifth is the final level of autonomous driving. The car is now completely guided by the system and performs all the necessary tasks automatically. Even complex situations - such as crossing an intersection, driving through a roundabout or the correct behaviour at a zebra crossing - can be handled by the autonomous car.
So far, however, there is no legal framework for fully automated vehicles. Therefore, the rights and obligations of the manufacturers of the cars/trucks, software developers and the insurance companies, in the automated mode of operation, are still completely unclear. The ADAC experts suggest only 3 operating modes instead of 5 levels (Paulsen, 2018), where at the moment the car supports us (1st operating mode), soon it will drive itself (2nd operating mode) and in future driverless (3rd operating mode). They can be described as follows:
First mode: assisted driving
The driver is always and at all times responsible for driving. As far as possible, the vehicle keeps in lane, brakes and accelerates. But: feedback is not mandatory and a sudden stop is always possible.
Second mode: automated driving
The vehicle only drives itself in the application case specified by the manufacturer (e.g. stop-and-go in a traffic jam). The driver may temporarily turn away from the driving task and the traffic situation. Outside employment is possible. The driver is only liable if he has not complied with the request to take over.
Third mode: autonomous driving
The driver becomes a passenger, or the vehicle can be operated without occupants. The autonomous mode can be limited to defined routes. An operator (not the driver) must constantly monitor the vehicle in order to be able to react to operational problems (flat tyres, etc.).
In a forecast of the introduction of automation functions in the passenger car fleet by 2050 in Germany, 3 different automation functions are considered that build on each other (Altenburg et al., 2018):
- Motorway pilot: The motorway pilot enables level 4 highly automated driving on all motorways. This automation level enables the vehicle to move on this type of road completely without monitoring or even intervention by the driver. When leaving the motorway, the vehicle hands control back to the human driver.
- City Pilot: The City Pilot can take control not only on motorways, but also in the entire urban environment up to a speed of 50 km/h. On rural roads that do not have a structural separation of the directional lanes and that are not subject to the urban speed limit, the driving task must be taken over by a human.
- Door-to-door pilot: This most far-reaching automation function allows vehicles to drive the entire road network in automated mode without driver intervention. Here, too, automation will encompass level 4.
González-González et al. (2020) summarise the following goals for urban planning in their article on parking in the future (preparing European cities for the advent of automated vehicles):
- Objective 1 - Promote social justice and inclusiveness (safeguard the city’s core social values).
- Objective 2 - Reduce the need for mobility (prevent urban sprawl, unsustainable use of land and resources and the increase in circulating single occupant vehicles).
- Objective 3 - Promote active mobility (walking and cycling are critical to a healthy society and are compromised by the provision of door-to-door services).
- Objective 4 - Promote high quality multimodal public transport system (To counteract the substitution of public transport by individual PAVs or SAVs and thus the increase of vehicles on the roads and the associated energy and land consumption).
- Objective 5 - Reduce the number of circulating vehicles (e.g. by releasing large areas of land within the city to be converted into new attractive, high quality and liveable areas).
- Objective 6 - More public, high-quality urban space for citizens (ensuring inclusive public spaces, renaturalising urban areas, improving facilities and their accessibility, making the city centre more attractive than the periphery, and avoiding segregation)
- Objective 7 - Redensification, regeneration and renewal of core areas (Releasing large areas of parking could support cultural identity. Creating more cycling and walking routes, green spaces and necessary urban facilities would also contribute to these objectives).
- Objective 8 - Avoid VMT growth and sprawl (Sprawl is described as one of the most inefficient and unsustainable urban development patterns due to its high land and resource consumption).
- Objective 9 - Safety (The transition period of AV implementation could be supported by different types of measures, such as reducing the need for mobility and circulating vehicles).
For the AV implementation process in urban planning, González-González et al. (2020) propose the following 3 strategies:
“Safe and shared transition” aims to ensure safe coexistence between AVs and other mobility options (i.e. conventional vehicles, pedestrians and cyclists) during the implementation phase and to promote the adoption of SAVs over PAVs, mainly through regulatory measures related to access and parking restrictions and lane demarcation.
“Shared and active mobility” aims to promote high-quality and sustainable public transport and a shift away from individualistic forms of mobility through infrastructure-related measures such as improving public transport services, more connections to key attractive hubs, market-related measures such as incentives for businesses, higher charges for single-occupancy vehicles, and regulatory measures such as relocation of work centres.
“Urban reclaiming” aims to improve the fabric of new urban areas by redefining urban space with the aim of reusing street space and parking, for example through regulatory measures (e.g. reallocating parking spaces, reducing lanes) and providing new public amenities, high-quality public green spaces, sporting or cultural areas. At the same time, the third way seeks to avoid urban sprawl through regulatory or market-based measures such as minimum density standards or kilometre-based taxes.
Key stakeholders
- Affected: Citizens, drivers, cyclists, public transport users and drivers, pedestrians
- Responsible: National governments, City government, Car manufacturers
Current state of art in research
The main focus in research is currently concentrated on safety, social acceptance and external effects. Much of the ongoing research is considered to be privately owned by car manufacturers regarding safety and efficiency.
Although fully automated cars are seen as a means of improving network capacity and network reliability, making car use more accessible to a wider range of people and reducing accidents, several researchers warn that the rebound effect is that they could lead to a significant increase in car use, with a simultaneous decrease in pedestrians, cyclists and public transport, and that this could more than offset the benefits of increased capacity and lead to urban sprawl. May et al. (2020), following a review of the literature and qualitative analysis using causality diagrams, suggest that the key triggers of such change will be the market share of automated vehicles, whether private or shared, the extent to which capacity is increased, the potential to reduce time spent on parking and access, the potential reduction in the value of time spent in vehicles, and the expansion of the number of people who can drive. Their findings indicate that the introduction of automated cars is likely to have negative impacts on the environment, accessibility, health, urban sprawl and overall sustainability. They also suggest that these negative impacts can be mitigated by requiring that automated vehicles are provided in cities as shared vehicles rather than private vehicles. However, it seems that the fee needs to be higher than the one currently charged by car sharing companies to effectively avoid these negative impacts.
Zhou et al. (2020) investigates preference heterogeneity in mode choice for car-sharing and shared automated vehicles. They show that the use of AVs in car-sharing schemes does not currently receive much research focus, mainly due to the novelty of these technologies. Although the emergence of AVs could help drive car-sharing adoption by removing various barriers such as lack of parking and undesirable access costs. Proponents argue that the combination of carsharing and AVs (shared automated vehicles [SAVs]) offers unique opportunities for policy makers to positively influence and shape outcomes for society, for example SAVs could provide safe and accessible mobility services for the elderly, non-drivers, disabled and children (Howard, 2014; Yang & Coughlin, 2014).
Nordhoff et al. (2020) investigated the public acceptance of partially automated (SAE Level 3) passenger cars by means of a questionnaire among 9,118 drivers in eight European countries within the European L3Pilot project. Respondents considered partially automated cars easy to use, but were less likely to consider buying one. Slightly less than the majority could imagine engaging in off-road activities, such as talking to fellow drivers, surfing the Internet, watching videos or TV shows, observing the landscape or working. The positive effects of hedonic motivation, social influence and performance expectancy on the behavioural intention to use conditionally automated cars suggest that the benefits of conditionally automated cars need to be clearly demonstrated and promoted by public (e.g. media, policy) and private decision makers (e.g. manufacturers) through established communication channels and in social networks. Car dealers could be trained as real experts on automated driving functions to explain the strengths and limitations of the systems and to show the systems to customers, e.g. by offering test drives. Social networks (both online and offline) could play a more important role in promoting the benefits of conditionally automated cars, as friends, family and colleagues are an important and trusted source of information. Public authorities could form alliances with private organisations (e.g. automobile clubs, road construction companies) to launch joint awareness campaigns on the benefits of automated cars and to conduct test drives to familiarise the general public with automated cars.
Kaye et al. (2020) also examines the a priori acceptance of highly automated cars through the Theory of Planned Behaviour (TPB) and the Unified Theory of Acceptance and Use of Technology (UTAUT) in Australia, France and Sweden. For France and Sweden, participants who had prior knowledge of AVs reported significantly higher intentions to use automated cars in the future than those who had no prior knowledge. However, it is important to note that the majority of individuals in each country (i.e. over 80% of participants in Australia and France and 99% of participants in Sweden) reported pre-existing knowledge about AVs. In addition, individuals residing in France indicated a significantly greater intention to use highly automated vehicles if they were publicly available than individuals residing in Australia and Sweden. While it is not known why participants in France indicated significantly greater intentions to use automated cars when they are publicly available than participants in Australia and Sweden, these results support previous research that has shown that perceptions of AVs can vary across countries (e.g. Schoettle & Sivak, 2014) and highlight the need to consider country of residence when assessing AV uptake.
Vlakveld et al. (2020) investigates cyclists’ intentions to yield to automated cars at intersections when they have the right of way using an experiment with high-quality video animations. The results indicate that when cyclists have the right of way at intersections, they still sometimes swerve for cars. They swerve more often when the approaching car is an automated car than when the approaching car is a conventional car. However, when automated cars communicate to cyclists that the system has noticed them and that the car will act in accordance with traffic rules, cyclists tend to swerve less often than in similar conflict situations with a traditional car. It is important to note that the fact that cyclists are more inclined to continue when the automated car can indicate its intention to swerve does not necessarily improve road safety. It is beneficial for road safety if road users always correctly predict what other road users will do. Since the driving behaviour of automated cars can differ from the driving behaviour of cars driven by humans, indicating their intentions can help other road users to make the correct predictions because they know what to expect. However, this will only improve road safety if the displayed intentions are always correct.
Galich & Stark (2021) investigated the impact of the introduction of automated vehicles in private car ownership. The results show that a reduction or increase in private car ownership, depends to a large extent on the actual implementation of automated vehicles in terms of pricing structures, legal regulations, mobility services, etc. Despite the significantly higher waiting times and operating costs that other studies predict for less densely populated areas, the results showed that residents of rural areas are quite willing to accept higher costs and longer waiting times than their urban counterparts if they are offered reliable mobility alternatives to their own car. Rural residents were even willing to book automated ride-sharing services up to a day in advance for certain trips, such as commuting to work. Interviewed participants stated that they are willing to send their children to kindergarten or school alone in an automated vehicle, but that there should be kindergarten staff or school teachers to pick up the children from the vehicle and accompany them for the last few metres. Another very important point of the study is that automated vehicles could be a very attractive option for older people or people with mobility impairments and thus create a new social group of car owners. This could be socially desirable, as the mobility needs of this group often cannot be met by the currently available means of transport. However, this new social group of car owners can have a negative impact on land use, hence, it is still more favourable that public transport operators and car and ridesharing providers consider offering special services that address the specific needs of this user group. In this way, the mobility needs of this group could be met without leading to an increase in car ownership.
Current state of art in practice
Successes in research and development of automated vehicles differ from practical developments and reality on the roads. A recent study by the Prognos Research Institute on automated driving for the ADAC shows that automated driving will only slowly become established. This is mainly due to the fact that cars are in use for up to twenty years on average, and new technologies increase their presence very gradually in the overall stock. According to the forecast, the share of new vehicles in which the driver can completely turn away from the driving task on all motorways will increase in the “optimistic” case from 2.4 % in 2020 to as much as 70 % in 2050. From 2030 onwards, cars with Citypilot, i.e. the ability to drive alone both on the motorway and in the city, will gradually appear on the roads. And only after 2040 will a larger number of cars be available that can go from door to door fully autonomously, i.e. that no longer need a driver even on country roads. This means that for a long time to come, completely normal vehicles will be on the road alongside fully automated ones. This also puts the hope of rapid safety gains through fully automated cars in the next few decades. The fact that fewer and fewer people will die or be injured in road traffic will be due to the spread of efficient assistance systems, such as the emergency brake assistant (Rudschies & Kroher, 2020).
At the forecast horizon of 2050, about half of the vehicles will already have an automation function, but in most cases this will only be usable on motorways. Significant penetration of vehicles capable of automated driving throughout the network is not expected until after 2050. Vehicles with automation features are likely to account for significant shares of driving by 2050, but the limitations of the driving features delivered, mean that only a fraction of this will take place on road types for which suitable automation is also available. In addition, it cannot be assumed that the functions will be fully deployed immediately. Consequently, by 2050 a maximum of one in five vehicle km could be provided by automation. This value varies greatly between the different types of roads: while on motorways a good 40% of the mileage could be automated, on rural roads it is still less than 4%. The low proportion of automated driving also influences the safety effects. Automated driving can only make significant contributions slowly towards the end of the forecasted horizon. Since serious consequences of accidents occur particularly strongly on rural roads, where automation will hardly have any effect by 2050, the effect of automation on traffic fatalities will still be marginal by that time (Altenburg et al., 2018).
Driverless cars are to be able to participate in road traffic in Germany as early as 2022. Fully automated motor vehicles of the so-called “level four” will then be allowed to travel on certain defined routes on public roads. In fully automated driving, the computer can take complete control of the car in certain applications without being monitored by a human driver. In emergencies, the system should also bring the vehicle to a halt at the side of the road. According to the German Ministry of Transport, this technology could be used for shuttle services or freight transport, for example (Rudschies & Kroher, 2020).
The competition for the best technology for fully automated driving is currently ongoing worldwide. According to VW boss Herbert Diess, however, German car manufacturers are “one to two years behind” in developing the technology for fully automated driving. At the forefront of know-how, almost all experts locate companies in the USA - especially the US company Waymo, which belongs to Google. In the US state of Arizona, Waymo has been running robot cars as taxis for a while now - but still with a safety driver behind the wheel. Customers can order their ride via app and are picked up wherever they happen to be. On average in the vehicles run by Waymo the security guard only has to intervene 0.09 times per 1000 miles driven.
The development gap between Germany and the Americans could be due to the fact that German car manufacturers work with a higher degree of thoroughness. Christian Weiß, system developer at Daimler, emphasises: “Basically, safety comes before speed for us.” For him, the Tesla accidents are a good example of what can happen when immature technology is used in production vehicles. Daimler expert Timo Winterling adds: “To understand a scene, we have to get the last out of the sensors.”
In Europe, BMW is currently heavily involved in collecting and analysing data in real traffic. At the BMW Campus in Unterschleissheim near Munich, which was founded specifically for this purpose, around 1700 specialists are now working on developing the necessary software algorithms for highly automated driving. As test vehicles, 40 BMWs are on the road collecting huge amounts of data and images in road traffic. For storage, BMW has built two data centres with a capacity of 500 petabytes (PB), a storage size that would hold all the words ever written and printed in human history about five times over (Rudschies & Kroher, 2020). Moreover, currently operating test sites across Europe have been gathered under connectedautomateddriving.eu.
Relevant initiatives in Austria
In December 2016, a legal framework for automated vehicles and their systems was created in Austria. According to this, test drives by research institutions and vehicle manufacturers were allowed to assign certain driving tasks, such as keeping a distance, accelerating, braking or keeping in lane, to an assistance system or automated or connected driving system present in the vehicle, in compliance with the other legal regulations.
Since 11 March 2019, hands-free driving on motorways and with ‘motorway assistant with automatic lane guidance’ and automatic parking with parking assistant have been permitted. “However, one must be able to intervene immediately if an unexpected situation arises. Therefore, distracting activities, such as operating a mobile phone, remain prohibited. The system may also not be used in construction areas.
Truly autonomous driving is currently only allowed in Austria in test operation on certain stretches of road and requires a separate permit. This is primarily to allow companies to test self-driving cars.
With the action package Automated Mobility (2019-2022), the government programme 2017-2022 has set out to develop Austria into a pioneering country and thus also into a research, development and production location for automated driving in close cooperation with the automotive industry and research. In particular, the ministry will continue to promote test tracks and related research projects.
Impacts with respect to Sustainable Development Goals (SDGs)
Impact level | Indicator | Impact direction | Goal description and number | Source |
---|---|---|---|---|
Individual | Potential for increasing (access to) mobility in elderly and other disadvantaged groups | + | Equality (5,10) | Howard, 2014; Yang & Coughlin, 2014 |
Individual | More usable time due to automatization of driving tasks | + | Sustainable economic development (8,11) | Rudschies & Kroher, 2020 |
Systemic | Potential for reduction in accidents | + | Health & Wellbeing (3) | Rudschies & Kroher, 2020 |
Systemic | Potential for increase in car ownership | - | Environmental sustainability (7,12-13,15) | May et al., 2020 |
Open questions
- Can ownership of automated cars satisfy the mobility needs of a multi-person household that currently relies on two, three or even more cars?
- Who is responsible if the technology in semi-automated mode violates a speed limit or other traffic regulations?
References
- Altenburg, S., Kienzler, H.-P., & Maur, A. A. der. (2018). Einführung von Automatisierungsfunktionen in der Pkw-Flotte.
- European Commission. (2019). Automated road transport.
- Galich, A., & Stark, K. (2021). How will the introduction of automated vehicles impact private car ownership? Case Studies on Transport Policy, 9(2), 578–589. https://doi.org/10.1016/j.cstp.2021.02.012
- Howard, D. (2014). Public Perceptions of Self-driving Cars: The Case of Berkeley, California. MS Transportation Engineering, 2014(1), 21.
- Kaye, S. A., Lewis, I., Forward, S., & Delhomme, P. (2020). A priori acceptance of highly automated cars in Australia, France, and Sweden: A theoretically-informed investigation guided by the TPB and UTAUT. Accident Analysis and Prevention, 137, 105441. https://doi.org/10.1016/j.aap.2020.105441
- May, A. D., Shepherd, S., Pfaffenbichler, P., & Emberger, G. (2020). The potential impacts of automated cars on urban transport: An exploratory analysis. Transport Policy, 98, 127–138. https://doi.org/10.1016/j.tranpol.2020.05.007
- Nordhoff, S., Louw, T., Innamaa, S., Lehtonen, E., Beuster, A., Torrao, G., Bjorvatn, A., Kessel, T., Malin, F., Happee, R., & Merat, N. (2020). Using the UTAUT2 model to explain public acceptance of conditionally automated (L3) cars: A questionnaire study among 9,118 car drivers from eight European countries. Transportation Research Part F: Traffic Psychology and Behaviour, 74, 280–297. https://doi.org/10.1016/j.trf.2020.07.015
- Paulsen, T. (2018, November 7). Autonomes Fahren: 5 Level zum selbstfahrenden Auto | ADAC. ADAC. https://www.adac.de/rund-ums-fahrzeug/ausstattung-technik-zubehoer/autonomes-fahren/grundlagen/autonomes-fahren-5-stufen/
- Rudschies, W., & Kroher, T. (2020, May 21). Autonomes Fahren: Der aktuelle Stand der Technik | ADAC. 20.11.2020. https://www.adac.de/rund-ums-fahrzeug/ausstattung-technik-zubehoer/autonomes-fahren/technik-vernetzung/aktuelle-technik/
- Schoettle, B., & Sivak, M. (2014). A survey of public opinion about autonomous and self-driving vehicles in the US, UK and Australia. UMTRI, Transportation Research Institute, July, 1–38.
- Vlakveld, W., van der Kint, S., & Hagenzieker, M. P. (2020). Cyclists’ intentions to yield for automated cars at intersections when they have right of way: Results of an experiment using high-quality video animations. Transportation Research Part F: Traffic Psychology and Behaviour, 71, 288–307. https://doi.org/10.1016/j.trf.2020.04.012
- Yang, J., & Coughlin, J. F. (2014). IN-VEHICLE TECHNOLOGY FOR SELF-DRIVING CARS: ADVANTAGES AND CHALLENGES FOR AGING DRIVERS J. International Journal of Automotive Technology, 15(2), 333−340.
- Zhou, F., Zheng, Z., Whitehead, J., Washington, S., Perrons, R. K., & Page, L. (2020). Preference heterogeneity in mode choice for car-sharing and shared automated vehicles. Transportation Research Part A: Policy and Practice, 132, 633–650. https://doi.org/10.1016/j.tra.2019.12.004
8.2 Parking infrastructure for automated vehicles
Updated: 29th August 2022
Synonyms
Automated vehicles (AVs), Shared AV (SAV), Private ownership AV (PAVs), Smart Parking (SP), Automated Valet Parking (AVP), Short-range Automated Valet Parking (SAVP), Long-range Automated Valet Parking (LAVP)
Definition
Automated vehicles (AVs) will undoubtedly have a major impact on urban form and development patterns. Literature on the impact of AVs points to both major opportunities as well as threats. Optimistically, AVs could reduce parking needs, traffic volumes and street space, especially if they are shared. This could enable the redesign of these spaces, leading to densification and an improvement in the attractiveness of city centres (Alessandrini et al., 2015; Heinrichs, 2016; Milakis et al., 2017; Zhang et al., 2015; González-González et al., 2020). Conversely, however, the flexibility, convenience and reduction in the value of travel time offered by AVs could lead to an increase in distance travelled and an intensification of urban sprawl (Cohen et al., 2017; Milakis et al., 2017; Zakharenko, 2016; González-González et al., 2020).
Currently, a vehicle remains parked for an average of 95% of its lifetime (Cogill et al., 2014). To accommodate the increasing number of vehicles, a large space is usually used for parking in urban areas (Zhou et al., 2018). Parking locations and spaces are usually selected in comparison to the human-vehicle ratio. For example, in San Francisco, about 31% of the total space is used for parking, in London 16%, in New York 18% and in Los Angeles 81% (Manville & Shoup, 2005; Pierce & Shoup, 2013). The integration of Internet of Things (IoT) with cloud computing enables the development of automated valet parking and smart cities (Huang et al., 2018). These services include real-time processing, location awareness, data and load management for environmental sensing and coordination (Khalid et al., 2018; Ni et al., 2019).
The advantages of Smart Parking are the precise navigation and consequently lower fuel consumption for parking. Moreover, the unnecessary driving around in search of parking spaces can be avoided if information about the availability of parking spaces in a car park is at hand in advance (Khalid et al., 2021; Liu et al., 2019; Rajabioun & Ioannou, 2015).
Automated parking (AVP) is the term used when an AV drops the user off at a predefined drop-off point and performs route selection towards the selected parking space and the parking process in an automated manner (Williams, 2019).
AVP can be divided into Short-range Automated Valet Parking (SAVP) and Long-range Automated Valet Parking (LAVP) (Khalid et al., 2021). In SAVP, the user parks the AV at the entrance of the car park. The AV scans the available parking spaces using advanced vision techniques, avoids obstacles using sensor technologies, and is parked in the designated parking space using automated vehicle manoeuvring techniques (Lou et al., 2019; Wang et al., 2015). Furthermore, SAVP can be performed in multi-storey car parks using advanced localisation techniques (Haas et al., 2020).
In Long-range Automated Valet Parking (LAVP) in comparison, the user can drop off the AV in the city centre (or at a specific drop-off point), whereupon the AV will drive with the appropriate route from the drop-off point to the car park (Khalid et al., 2021).
In the future, cities should adapt to the introduction of AVs, but AVs should also be developed to meet the expectations of ideal future cities (Porter, 2018). In any case, on the part of local governments and planners, work should begin to prepare adaptable legal, urban planning and land use regulations to accommodate such a disruptive mode of transport as AVs (Cohen & Cavoli, 2019; Legacy et al., 2019).
A general smart parking model consists of the following elements (Kuran et al., 2015 in Khalid et al., 2021):
- Parking module ensures and defines all operations within the car park (e.g. scanning parking spaces, updating information for the vehicle in real time).
- User interface connects the driver to the manager module and the parking servers. (Checking real-time information on available parking spaces, reserving a parking space, processing payment for a reserved space and route instructions from the parking module).
- Communication module is responsible for information exchange, encryption, error control and reliability assessment.
- Parking Manager handles data management on reserved parking spaces as well as the user’s transaction record for each occupied space.
- Parking Lot Controller detects whether a particular space is free or occupied.
Compared to traditional parking, Smart Parking provides drivers with pre-parking information (e.g. location, availability of parking spaces and fees) and offers the possibility of pre-registration and real-time booking.
Similar to the levels of automation of cars, there are different levels of automated parking (Chirca et al., 2015; Khalid et al., 2021, 2018):
- Level 1: The driver must fully supervise the parking process
- Level 2: The driver can remain outside the vehicle to monitor and control the entire parking process via smart devices such as mobile phones.
- Level 3: Advanced sensor and 3D mapping technologies are used to avoid obstacles and find the best route to the parking space (Chirca et al., 2015).
- Level 4: The driver leaves the AV at the car park entrance and it navigates autonomously to a free parking space.
Key stakeholders
- Affected: Users of automated cars
- Responsible: Carpark Companies, City governments, Car manufacturers, City planners
Current state of art in research
Milakis et al. (2017) optimistically estimated that AVs could lead to a reduction in parking demand of between 67 and 90 %. Zhang (2017) estimated a reduction of at least 20 parking spaces per SAV due to the reduction of vehicles and an increase in vehicle occupancy. Perhaps even more could be reduced by increasing the efficiency of parking automation. Car parks could increase their capacity by up to 60% as aisles, ramps and doorways would no longer be needed (Heinrichs, 2016). On the one hand, the spatial distribution of parking spaces could be distributed in attractive parts of high-density city centres, or out-of-centre parking spaces could accommodate around 97% of daily parking demand (Zakharenko, 2016), as AVs could pick up and drop off passengers at different points within the city and park far away when not needed (González-González et al., 2020). However, this would likely lead to an increase in travel distance and frequency due to changing travel costs and the value of passenger time. Fagnant & Kockelman (2015) estimated an increase in vehicle kilometres travelled (VMT) of up to 26% following a 90% implementation of AVs. Milakis et al. (2017) estimate an increase of between 1-23% by 2030 and between 10-71% by 2050 in VMT for the Netherlands. Furthermore, several regional transportation planning organisations in the US projected an increase of 5-20% (Guerra, 2016). However, Soteropoulos et al. (2019) point to a reduction in VMT of around 10-25 % if a large proportion of travellers choose ridesharing.
When talking about smart car parking as IoT Systems we can distinguish between different forms and architectures. The figure below gives an overview of different approaches to smart parking, starting with smart parking systems (grey), sensors used in smart parking systems (orange), networking technologies (blue), user interfaces (yellow), computational approaches (grey) and provided services (yellow) (Fahim et al., 2021; Winter, 2021). High priority in research has been given to parking supervision, while gate management receives least priority.
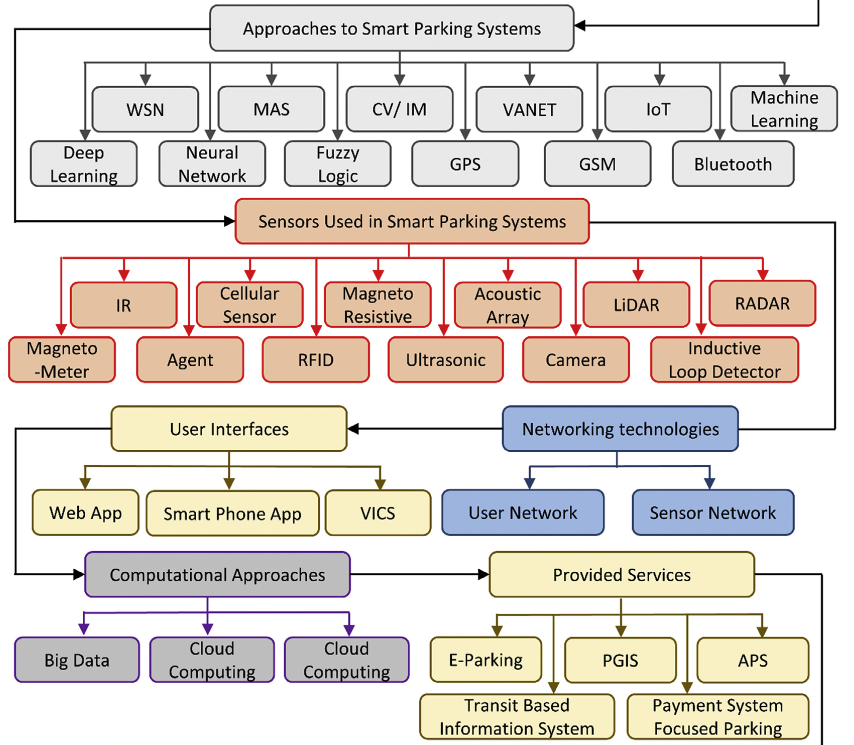
Figure 8.1: Smart Parking (Fahim et al., 2021, p.2)
Levin et al. (2020) conclude in their paper on parking infrastructure design for repositioning fully automated vehicles that empty AV repositioning trips (due to lower prices in more distant parking spaces) contribute to network congestion. They have developed a traffic assignment model with endogenous parking choice (where fuel consumption depends on traffic congestion). Since parking choice depends on fuel consumption and parking fees, urban planners can influence reallocation trips by adjusting parking fees. Analysis shows that the genetic algorithm was effective in finding parking options that reduce the impact of empty trips on congestion and reduce the cost of building parking infrastructure in zones with high land values. A similar study by Jiménez et al. (2022) on perception, positioning and decision-making algorithms for autonomous valet parking using the LiDAR sensor tested in a real parking lot showed promising results with accurate behaviour of the AV. Further studies analyse various autonomous parking systems in different areas (e.g. urban space, on-street and off-street parking) and with different network positioning. They mostly demonstrate successful technological functionality, while further practicability still needs future testing (Plihal et al., 2022).
Khalid et al. (2021) summarises the future challenges of SP and AVP as follows:
- Data protection and security
- Secure communication (V2V and V2I)
- Data protection
- Congestion avoidance
- Routing (the shortest route is not always optimal, as other factors such as price, infrastructure and congestion can also be taken into account depending on the objective)
- Parking space utilisation (parking space utilisation can be increased but parking will be challenging for normal drivers in car parks for AVs)
- 3D localisation maps (in most car parks the global positioning system (GPS) is not able to locate a free parking space exactly)
- Deployment
- Drop-off and pick-up spot
- Car park (in remote areas or on the outskirts of the city centre to avoid traffic congestion)
- Scheduling
- Car park scheduling (novel car park scheduling algorithm is needed that takes into account user demand and real-time street information)
- Vehicle sharing (to further reduce congestion)
- Charging scheme (efficient charging solutions are required in the planning process to support vehicles, especially in fully automated mode)
- Green parking (improving parking search capacity and reducing journey time can help reduce carbon emissions and pollution)
- Accessibility (reservation, convenient ride, economic/dynamic pricing, hassle-free drop-off and pick-up experience, and green planning and scheduling algorithms)
- Integrated parking system (degree of integration of various components; built-in GPS, localisation maps of car parks, reservation algorithm, secure payment system and real-time monitoring into the future system)
- Digitised car parks (With proper management, the number of cars on the road can be reduced and green transport can be realised)
Current state of art in practice
The world’s first fully automated and driverless car park with SAE Level 4 parking function was officially approved in July 2019 at the Mercedes-Benz Museum in Stuttgart. This project was made possible by Bosch and Daimler. The automated drive-up and parking service is accessed via smartphone app and does not require a driver at all. The necessary intelligent parking infrastructure, which supports the mixed traffic of AVP and manually controlled vehicles, comes from Bosch. The technically equipped vehicles come from Mercedes-Benz. Together, the two partners defined the interface between infrastructure and vehicle and adapted the sensor technology and vehicle software accordingly. Due to the sensor technology used in the infrastructure, the necessary equipment of a vehicle is essentially limited to an automatic transmission, ESP, electric parking brake and steering assistance, a start/stop function and the communication unit. The car park system is based on the interaction of intelligent infrastructure and vehicle technology. The necessary car park infrastructure includes the required sensor technology and IT technology to calculate the driving routes and to fulfil all requirements regarding safety. A technical unit that communicates with the vehicle and a cloud connection for interaction with the backend are also required (Robert Bosch GmbH, 2021a). Garages with AVP could have 20% more capacity, as no space needs to be left for the occupants to exit (Ehrenfeuchter, 2020; Robert Bosch GmbH, 2021a).
Further, AVP garages are planned at Stuttgart Airport and in Detroit (Robert Bosch GmbH, 2021b). Tests have already been carried out in Detroit with Ford Escape test cars (Of-Allinger, 2020). In contrast to earlier test projects, Bosch no longer uses expensive and prominent lidar sensors in the multi-storey car park at Stuttgart Airport, but low-cost cameras. Around 180 of them were mounted on the ceiling. They determine the position of the vehicle ten times per second. A server determines the data and the driving command for the car is calculated and transmitted to the car via Wifi. Thanks to a specially speckled coating, the system also detects possible objects on the floor. If a luggage trolley is in the way, the technology reports the incident and informs an employee. Additionally, in July 2021, Hong Kong revealed its first automated robotic parking system in which robots park vehicles on pallets that then transport the car to a vacant parking space (N. N., 2021).
The Mercedes S-Class is the first series-produced vehicle in the world with AVP technology on board. No additional sensors are required compared to the existing standard technology, but only a special module is necessary to communicate with the car park computer. Potential buyers will be able to order the comfort function in future via the special equipment “Intelligent Park Pilot”. According to the manufacturer, the costs for this function are in the low four-digit range.
How quickly the technology will spread and how well it will be accepted remains to be seen. However, the advantages are that the sensor technology is not required to be in the car, but rather in the parking garage. This makes it easier for other car manufacturers to use the technology. Bosch is already in intensive talks with other companies. Since the camera infrastructure can simply be installed in a multi-storey car park, the technology will also be usable for older buildings. Modular retrofitting, i.e. floor by floor, is also possible (Ehrenfeuchter, 2020).
Impacts with respect to Sustainable Development Goals (SDGs)
Impact level | Indicator | Impact direction | Goal description and number | Source |
---|---|---|---|---|
Individual | Increased comfort and time saving | + | Health and wellbeing (3) | Robert Bosch GmbH (2021b) |
Systemic | Unpredictable effects on pollution and traffic congestion | ~ | Environmental sustainability (7,12-13,15) | Akhavan-Rezai et al., 2018; Khalid et al., 2019 |
Systemic | Increased collaborations between technology companies and car manufacturers | + | Partnership & collaborations (17) | Ehrenfeuchter, 2020; Of-Allinger, 2020; Robert Bosch GmbH, 2021b |
Open questions
- How well will the technology be adopted?
- How well will the mix work with AVs and manually driven cars in parking infrastructure?
- How error-prone will such parking systems be?
References
- Akhavan-Rezai, E., Shaaban, M. F., El-Saadany, E. F., & Karray, F. (2018). New EMS to incorporate smart parking lots into demand response. IEEE Transactions on Smart Grid, 9(2), 1376–1386. https://doi.org/10.1109/TSG.2016.2587901
- Alessandrini, A., Campagna, A., Site, P. D., Filippi, F., & Persia, L. (2015). Automated vehicles and the rethinking of mobility and cities. Transportation Research Procedia, 5, 145–160. https://doi.org/10.1016/j.trpro.2015.01.002
- Chirca, M., Chapuis, R., & Lenain, R. (2015). Autonomous Valet Parking System Architecture. IEEE Conference on Intelligent Transportation Systems, Proceedings, ITSC, 2015-Octob, 2619–2624. https://doi.org/10.1109/ITSC.2015.421
- Cogill, R., Gallay, O., Griggs, W., Lee, C., Nabi, Z., Ordonez, R., Rufli, M., Shorten, R., Tchrakian, T., Verago, R., Wirth, F., & Zhuk, S. (2014). Parked cars as a service delivery platform. 2014 International Conference on Connected Vehicles and Expo, ICCVE 2014 - Proceedings, 138–143. https://doi.org/10.1109/ICCVE.2014.7297530
- Cohen, T., & Cavoli, C. (2019). Automated vehicles: exploring possible consequences of government (non)intervention for congestion and accessibility. Transport Reviews, 39(1), 129–151. https://doi.org/10.1080/01441647.2018.1524401
- Cohen, T., Jones, P., & Cavoli, C. (2017). Social and behavioural questions associated with autonomous vehicles. January, 1–124.
- Ehrenfeuchter, M. (2020, October 14). Automated Valet Parking (AVP): Test am Flughafen Stuttgart | AUTO MOTOR UND SPORT. https://www.auto-motor-und-sport.de/tech-zukunft/mobilitaetsservices/automated-valet-parking-flughafen-stuttgart-s-klasse/
- Fagnant, D. J., & Kockelman, K. M. (2015). Dynamic ride-sharing and optimal fleet sizing for a system of shared autonomous vehicles. Transportation, 1, 1–16.
- Fahim, A., Hasan, M., Chowdhury, M. A. (2021). Smart parking systems: comprehensive review basen on various aspects. Heliyon. 7(5), e07050, 1-21. https://doi.org/10.1016/j.heliyon.2021.e07050
- González-González, E., Nogués, S., & Stead, D. (2020). Parking futures: Preparing European cities for the advent of automated vehicles. Land Use Policy, 91, 104010. https://doi.org/10.1016/j.landusepol.2019.05.029
- Guerra, E. (2016). Planning for Cars That Drive Themselves: Metropolitan Planning Organizations, Regional Transportation Plans, and Autonomous Vehicles. Journal of Planning Education and Research, 36(2), 210–224. https://doi.org/10.1177/0739456X15613591
- Heinrichs, D. (2016). Autonomous Driving and Urban Land Use BT - Autonomous Driving: Technical, Legal and Social Aspects (M. Maurer, J. C. Gerdes, B. Lenz, & H. Winner, Eds.; pp. 213–231). Springer Berlin Heidelberg. https://doi.org/10.1007/978-3-662-48847-8_11
- Huang, C., Lu, R., Lin, X., & Shen, X. (2018). Secure automated valet parking: A privacy-preserving reservation scheme for autonomous vehicles. IEEE Transactions on Vehicular Technology, 67(11), 11169–11180. https://doi.org/10.1109/TVT.2018.2870167
- Jiménez, F., Calvijo, M., Cerrato, A. (2022). Perception, Positioning and Decision-Making Algorithms Adaptation for an Autonomous Valet Parking System Based on Infrastructure Reverence Points Using One Single LiDAR. Sensors. 22(3), 979. https://doi.org/10.3390/s22030979
- Khalid, M., Cao, Y., Aslam, N., Raza, M., Moon, A., & Zhou, H. (2019). AVPark: Reservation and cost optimization-based cyber-physical system for long-range autonomous valet parking (L-AVP). IEEE Access, 7, 114141–114153. https://doi.org/10.1109/ACCESS.2019.2930564
- Khalid, M., Cao, Y., Aslam, N., Suthaputchakun, C., Arshad, M., & Khalid, W. (2018). Optimized Pricing Scheduling Model for Long Range Autonomous Valet Parking. 2018 International Conference on Frontiers of Information Technology (FIT), 65–70. https://doi.org/10.1109/FIT.2018.00019
- Khalid, M., Wang, K., Aslam, N., Cao, Y., Ahmad, N., & Khan, M. K. (2021). From smart parking towards autonomous valet parking: A survey, challenges and future Works. In Journal of Network and Computer Applications (Vol. 175, p. 102935). Academic Press. https://doi.org/10.1016/j.jnca.2020.102935
- Kuran, M. S., Carneiro Viana, A., Iannone, L., Kofman, D., Mermoud, G., & Vasseur, J. P. (2015). A smart parking lot management system for scheduling the recharging of electric vehicles. IEEE Transactions on Smart Grid, 6(6), 2942–2953. https://doi.org/10.1109/TSG.2015.2403287
- Legacy, C., Ashmore, D., Scheurer, J., Stone, J., & Curtis, C. (2019). Planning the driverless city. Transport Reviews, 39(1), 84–102. https://doi.org/10.1080/01441647.2018.1466835
- Levin, M. W., Wong, E., Nault-Maurer, B., & Khani, A. (2020). Parking infrastructure design for repositioning autonomous vehicles. Transportation Research Part C: Emerging Technologies, 120, 102838. https://doi.org/10.1016/j.trc.2020.102838
- Liu, Z., Xie, Y., Chan, K. Y., Ma, K., & Guan, X. (2019). Chance-Constrained Optimization in D2D-Based Vehicular Communication Network. IEEE Transactions on Vehicular Technology, 68(5), 5045–5058. https://doi.org/10.1109/TVT.2019.2904291
- Lou, L., Zhang, J., Xiong, Y., & Jin, Y. (2019). An improved roadside parking space occupancy detection method based on magnetic sensors and wireless signal strength. Sensors (Switzerland), 19(10). https://doi.org/10.3390/s19102348
- Manville, M., & Shoup, D. (2005). Parking, people, and cities. Journal of Urban Planning and Development, 131(4), 233–245. https://doi.org/10.1061/(ASCE)0733-9488(2005)131:4(233)
- Milakis, D., Snelder, M., Van Arem, B., Van Wee, B., & De Almeida Correia, G. H. (2017). Development and transport implications of automated vehicles in the Netherlands: Scenarios for 2030 and 2050. European Journal of Transport and Infrastructure Research, 17(1), 63–85. https://doi.org/10.18757/ejtir.2017.17.1.3180
- Milakis, D., Van Arem, B., & Van Wee, B. (2017). Policy and society related implications of automated driving: A review of literature and directions for future research. Journal of Intelligent Transportation Systems: Technology, Planning, and Operations, 21(4), 324–348. https://doi.org/10.1080/15472450.2017.1291351
- N. N. (2021). HKSTP reveals Hong Kong’s first automated robotic parking system at science park to advance smart city vision. HKSTP. Available at: https://www.hkstp.org/press-room/hkstp-reveals-hong-kong-s-first-automated-robotic-parking-system-at-science-park-to-advance-smart-city-vision/ [Accessed: 24 August 2022]
- Ni, J., Lin, X., & Shen, X. (2019). Toward Privacy-Preserving Valet Parking in Autonomous Driving Era. IEEE Transactions on Vehicular Technology, 68(3), 2893–2905. https://doi.org/10.1109/TVT.2019.2894720
- Of-Allinger, A. (2020, August 26). Automated Valet Parking von Ford, Bosch und Bedrock | AUTO MOTOR UND SPORT. https://www.auto-motor-und-sport.de/tech-zukunft/automated-valet-parking-bosch-ford-bedrock/
- Pierce, G., & Shoup, D. (2013). Getting the prices right. Journal of the American Planning Association, 79(1), 67–81. https://doi.org/10.1080/01944363.2013.787307
- Plihal, J., Nedoma, P., Sestak, V., Herda, Z., Aksjonov, A. (2022). Transport Automation in Urban Mobility: A Case Study of an Autonomous Parking System. Vehicles. 4(2), 326-343. https://doi.org/10.3390/vehicles4020020
- Porter, L. (2018). The autonomous vehicle revolution: Implications for planning. Planning Theory and Practice, 19(5), 753–778. https://doi.org/10.1080/14649357.2018.1537599
- Rajabioun, T., & Ioannou, P. (2015). On-Street and off-street parking availability prediction using multivariate spatiotemporal models. IEEE Transactions on Intelligent Transportation Systems, 16(5), 2913–2924. https://doi.org/10.1109/TITS.2015.2428705
- Robert Bosch GmbH. (2021a). Automated Valet Parking – schnell, sicher, fahrerlos | Bosch Global. https://www.bosch.com/de/stories/automated-valet-parking/
- Robert Bosch GmbH. (2021b). Bosch Automated Valet Parking. https://www.bosch-mobility-solutions.com/de/loesungen/parken/automated-valet-parking/
- Soteropoulos, A., Berger, M., & Ciari, F. (2019). Impacts of automated vehicles on travel behaviour and land use: an international review of modelling studies. Transport Reviews, 39(1), 29–49. https://doi.org/10.1080/01441647.2018.1523253
- Wang, D. Z., Posner, I., & Newman, P. (2015). Model-free detection and tracking of dynamic objects with 2D lidar. International Journal of Robotics Research, 34(7), 1039–1063. https://doi.org/10.1177/0278364914562237
- Williams, L. (2019). The whole story of parking: The world of parking is no longer stationary. Eng. Technol., 14(2), 56–61.
- Winter, S., Salil, G. (2021). Smart Parking in Fast-Growing Cities. Challenges and Solutions. TU Wien Academic Press. ISBN: 978-3-85448-045-7. DOI: 10.34727/2021/isbn.978-3-85448-045-7. Available at: https://repositum.tuwien.at/bitstream/20.500.12708/18055/1/Winter-2021-SMART%20PARKING%20IN%20FAST-GROWING%20CITIES-vor.pdf [Accessed: 22 August 2022]
- Zakharenko, R. (2016). Self-driving cars will change cities. Regional Science and Urban Economics, 61, 26–37. https://doi.org/10.1016/j.regsciurbeco.2016.09.003
- Zhang, W. (2017). the Interaction Between Land Use and Transportation in the Era of Shared Autonomous Vehicles: a Simulation Model. August, 147.
- Zhang, W., Guhathakurta, S., Fang, J., & Zhang, G. (2015). Exploring the impact of shared autonomous vehicles on urban parking demand: An agent-based simulation approach. Sustainable Cities and Society, 19, 34–45. https://doi.org/10.1016/j.scs.2015.07.006
- Zhou, D., Yan, Z., Fu, Y., & Yao, Z. (2018). A survey on network data collection. Journal of Network and Computer Applications, 116, 9–23. https://doi.org/10.1016/j.jnca.2018.05.004
8.3 Automated road freight
Updated: 26th August 2022
Synonyms
automated driving systems (ADS), combustion-powered heavy vehicles (CHV), battery electric heavy vehicles (BEHVs), cooperative and automated vehicles (CAVs)
Definition
The transport sector will soon experience a revolution in automated driving systems (ADS), as reported by Wadud et al. (2016) and Flämig (2016). Some benefits in terms of user experience, efficiency, safety, mobility, productivity, energy, environment and economy have been reported with ADS by many studies (Alessandrini et al., 2015; Anderson et al., 2016; Brown et al., 2014; Chan, 2017; Harper et al., 2016; Wadud, 2017; Kopelias et al., 2020 and Khan et al., 2019), although significant increases in road safety from highly or fully automated vehicles are not certain, as shown by Kalra & Paddock (2016).
The goals and motivations of automated driving for passenger cars and freight transport differ greatly (Wadud, 2017; Nowakowski et al., 2015). For passenger cars, the main motivations are user experience and the environment, while for freight, the main drivers are productivity and profitability. For example, the increase in the cost of a vehicle related to automation hardware is less important in freight transport due to the lower share of automation hardware costs in the total acquisition costs compared to those of passenger cars. In addition, ADS enable higher profitability in freight transport, mainly due to a reduction in labour costs as well as facilitated logistics and increased utilisation and efficiency (Wadud et al., 2016). In addition, driver costs decrease significantly with high or full automation of driving, leading to early adoption of ADS in the freight sector (Ghandriz et al., 2020a).
The levels of automation are currently divided into 5 levels (Paulsen, 2018):
First level: assisted driving
Individual assistance systems provide support for specific driving tasks. Assisted driving is already a reality in many cars today. (see automatic adaptive cruise control and automatic lane departure warning).
Second level: partially automated driving
With semi-automated driving, the car/truck can temporarily perform some tasks itself, without any human intervention. For this purpose, various individual systems are combined with each other, in this case, the automatic adaptive cruise control with the emergency braking and lane departure warning systems. Further level 2 functions include overtaking assistant, automatic parking.
Third level: highly automated driving
Highly automated cars/trucks (Level 3) can perform certain driving tasks autonomously and without human intervention, but only for a limited period of time and under suitable conditions specified by the manufacturer. Level 3 cars will probably be on the road on motorways first: There is no oncoming traffic there, the lane markings are usually in order, and the roads are continuously recorded as digital maps. Since 2017, there has also been a legal framework for Level 3 cars in Germany: as soon as the driver puts his car into highly automated mode, he is allowed to turn his attention away from the road traffic. This means that the driver is allowed to read the newspaper. However, if the system detects a problem and sends a signal, the driver must take over the wheel immediately.
Level four: fully automated driving
In the development departments of the large car companies, but also at Apple, Google or Uber, engineers and computer scientists are working flat out on the full automation of the car, i.e. level 4 on the way to autonomous driving. In this level, the technical systems carry out all driving tasks automatically, and the car can also cover longer distances without intervention. So far, however, there is no legal framework for fully automated vehicles.
Fifth level: autonomous driving
The fifth is the final level of autonomous driving. The car is now completely guided by the system and performs all the necessary tasks automatically. Even complex situations - such as crossing an intersection, driving through a roundabout or the correct behaviour at a zebra crossing - can be handled by the autonomous car.
For a regulatory framework, seamless and harmonised cross-border EU and national regulation of automated road transport is seen by the European Commission, 2019 as a key factor for market introduction. Common procedures for testing, validation and certification enable the standardisation of solutions. Standards should also apply to data exchange and communication solutions. Ethical, legal, environmental and safety aspects should be regulated. The liability and responsibility of each actor in the road system must be defined before the introduction of automated driving.
Key stakeholders
- Affected: Truck drivers, Freight forwarders, Delivery companies
- Responsible: National Governments, City government, Freight forwarders, Delivery companies
Current state of art in research
The reduction in fuel consumption achieved with ADS due to improved vehicle use and controlled energy management is only up to 10% for passenger cars compared to when a human driver is involved, depending on the traffic scenario (Mersky & Samaras, 2016). The reduction in fuel consumption achieved with ADS is likely to be higher in freight transport, considering that heavy vehicles can form platoons, which theoretically reduce the energy intensity of the following vehicle by up to 25% when the distance between vehicles reaches zero, according to (Wadud et al., 2016); while measurements showed average fuel savings of 8% and 15% for 10 m and 4 m distance of the trucks in the platoon, respectively, according to (Tsugawa et al., 2016). However, as the European Commission (2016) points out, heavy-duty vehicles contribute to about 25 % of CO2 emissions from road transport in Europe, which are rising due to increasing road freight transport despite improved fuel efficiency. These figures suggest that the efficiency improvements offered by ADS, although a profitable business, cannot lead to a reduction in CO2 emissions in the long term; therefore, a further reduction of emissions in the transport sector is needed, motivating the development of greener solutions (Ghandriz et al., 2020a).
In a slightly simplified study of ADS in heavy vehicles, a reduction in the total cost of ownership (TCO) of trucks of between 27% and 46% for battery electric heavy vehicles (BEHVs) and between 11% and 41% for combustion-powered heavy vehicles (CHVs) makes BEHVs more profitable than BEHVs with human drivers by a factor of four over longer distances. It was observed that Level 4-5 automated BEHVs tend to have lower optimal speeds than vehicles with human drivers. Furthermore, it was shown that reducing speed for safety reasons is less costly to implement for Level 4-5 automated BEHVs than for Level 4-5 automated CHVs; in many scenarios, low speeds up to 60 km/h even reduced the TCO. The reduction in TCO in Level 4-5 automated trucks was mainly achieved by eliminating driver pay (35%-55%), the increase of the operating time on the road and thus the reduction of the downtimes (0%-15%), increasing payload by eliminating the cab (1.2%-5%), and optimising the drive system (0%-20%) (Ghandriz et al., 2020a). All the data and figures obtained were made available in (Ghandriz et al., 2020b) and provide valuable information on the feasibility and profitability of a planned freight transport operation with automation and electrification for a specific use case.
Pribyl et al., (2020) are investigating the effects of lower CAV penetrations. According to this, even with a low number of CAVs on the roads, the impact on transport and environmental performance indicators will be significant. However, this integration needs to be combined with other measures and policies such as the promotion of alternative powertrains if EU emission targets are to be met. However, results have also shown that CAVs can negatively affect other traffic parameters and lead, for example, to delays and other traffic flow phenomena.
Shin et al., (2018) analyse some of the technical trends related to intermodal automated freight transport systems (ATFS) that aim to reduce greenhouse gases and particulate matter, minimise infrastructure costs and logistics costs, and alleviate traffic congestion. Countries such as the US, Germany, the Netherlands and Japan have been actively developing technologies for ATFS. Gan et al., 2021 capture the characteristics of the swarm intelligence of truck drivers as a solid basis for the design, control and management of the future driverless truck swarm robotics system. The detection of the short-term location of truck drivers in advance, especially for areas with high freight demand, and thus the optimal use of freight capacity on the motorway, is the key component of freight matching. For city-level forecasting, it can help to determine the city points that truckers will be driving to, making it easier to plan an optimal route for them from the city’s perspective. It can also predict the internal transport capacity of the city, predict the bottleneck caused by the truck and provide decisions for inner-city traffic management.
Mulholland et al., (2018) define a global assessment of the decarbonisation of road freight transport by 2050. Overall, global road freight transport in tonnes-kilometer (tkm) is projected to grow 2.4-fold over the period 2015-2050 in their Reference Technology Scenario (RTS), with developing countries accounting for most of the growth, in line with economic growth. Developing countries are projected to account for 75% of road freight transport in 2050. In order to reduce dependence on fossil fuels, three key requirements are proposed:
- Fuel economy standards (minimum efficiency)
- Support for comprehensive data collection and information exchange
- Promoting the use of alternative fuels and the vehicles that use them
Recent research developed models to optimise the routing of freight (Sharma et al., 2022) as well as combining various modes of transport (Dekhtyaruk et al., 2021), simulations of mixed traffic (Chaudhry, 2022) and driver behaviour and experiences around automated freight vehicle platoons (Yang et al., 2022; Paddeu and Denby, 2022). Nonetheless, there is still uncertainty expected with most of the research, depending on the used model, area and implementation characteristics. Increasing research however, points towards awareness to use automation for freight to increase safety and decrease carbon use, while further optimising routes, platooning and mixed traffic solutions.
Current state of art in practice
The initial introduction of fully automated driving in closed traffic systems (e.g. traffic in a system network) and manageable scenarios (e.g. motorway, airport grounds, ports) helps to overcome the reluctance towards the technologies as well as the lack of standardisation. The gradual introduction of platooning, starting with manned lead and follow vehicles, could build up the necessary acceptance among the population. It should be examined whether the simultaneous introduction of overhead electric trucks and thus the creation of a dedicated lane would make sense in terms of capacity and safety requirements (Flämig, 2016).
A European Commission, 2019 report contained many automated road projects, but only one deals specifically with freight transport:
- Ensemble (01/06/2018- 31/05/2021)
The project aims to support the implementation of multi-brand truck platooning on European roads, so that a single truck can form a platoon with any other truck.
Other projects may have approached the issue in a different way, but the focus was on all vehicles. For example:
- Headstart (01/01/2019- 31/12/2021)
The project aims to define test and validation procedures for specific functionalities of Connected and Automated Driving (CAD), including key technologies such as communication, cyber security and positioning. The tests will take place both in simulation and in the real world to validate safety and security performance.
- Levitate (01/12/2018- 30/11/2021)
The project will develop a wide-ranging assessment framework to evaluate the impact of connected and automated transport (CAT) on all aspects of transport and individual mobility, as well as at a societal level. The project addresses the needs of local authorities, regional authorities and national governments to prepare for the increasing use of connected and automated systems, to understand the implications for mobility policy and to take the most effective measures to achieve broader societal goals.
Furthermore, the European Commission (2022) presents many automated road projects as well as urban freight transport policies and research funding within the Horizon 2020 (H2020) research program (Lozzi et al., 2018). Research and projects specifically addressing automated freight transport includes only a handful, mainly dealing with logistics planning and scheduling as well as Galileo-based solutions while main research and projects deal with rail automation and automation in road transport in general (European Commission, 2022).
In the US four manufacturers that dominate the US long-haul truck market have all begun to look at a driverless future. In 2019-2021, each of them has signed contracts with one of the three leading developers of self-driving vehicles (Alphabet’s Waymo, TuSimple Holdings and Aurora Innovation). Aurora plans to sell its driverless technology as a service, similar to the way jet engine makers charge airlines for hours spent on the throttle. TuSimple and Waymo Via have similar plans and are also operating cargo services while they test their technology. None of the partnerships with manufacturers are exclusive. The flurry of activity around trucking reflects a growing consensus that self-driving cars are still a long way from reaching most roads, and that 18-wheel trucks might be a better application area to establish the technology. Long-haul trucks drive straight most of the time on highways without traffic lights, cyclists or pedestrians crossing the road (Boudway, 2021).
Self-driving vehicles could have an immense impact on logistics and supply chain management. This is because fully automated trucks, last-mile delivery robots and other AVs fit into an increasingly automated distribution process. The automation of high-volume freight routes has the potential to deliver huge efficiency gains in terms of improved safety, increased capacity, lower operating costs and reduced environmental impact. An analyst at Gartner predicts that while self-driving trucks won’t be commercially available until 2024 at the earliest, supply chain executives responsible for transportation should start preparing for a fully automated future now. After all, fully automated trucks are just one part to fully automating the entire supply chain, from fully automated inventory planning to fully automated shipping to fully automated distribution. In a report (March 2021), a market research firm predicted that fully automated trucks will be more widely deployed by 2030, accounting for more than 10% of new heavy-duty truck sales on the road. By 2028, one-fifth of the world’s countries will have active regulations allowing the legal operation of production-ready fully automated vehicles, up from zero in 2019 (Eddy, 2021).
Societal aspect
Despite the imminent technical possibility of implementation, society may not be quite ready for it and political opposition because of the potential for large-scale unemployment among commercial drivers is expected (Wadud, 2017). Whereas, according to the IRU (International Road Transport Union), the European road transport sector is facing the biggest shortage of professional drivers in decades in 2019. According to a survey, the reasons are: the lack of attracting women to work in transport (female drivers make up only 2 per cent of employees in the European transport sector), attracting young people to work in the industry, the poor image of the profession, poor working conditions and being away from home and family for too long. In addition, there is the demographic ageing of the workforce. The average age of a truck driver in Europe is 44 (Kulikowska-Wielgus, 2019).
In the US, federal agencies have filed plans with the Office of Management and Budget (OMB) for a survey study to assess public appetite for automated driving systems (ADS) in the trucking sector. However, the project, Trucking Fleet Concept of Operations (CONOPS) for Managing Mixed Fleets, has already received public pressure. While the Federal Motor Carrier Safety Administration (FMCSA) received only eight comments on the proposal when it was first requested in November, all opposed it. They cited the “potential loss of jobs for truck drivers and safety concerns arising from ADS testing in the real world” before enough simulator testing has been done. “People who rely on truck driving jobs will become unemployed and will no longer have a way to earn a living and provide for themselves and their families,” one comment stated. If OMB approves the survey, a questionnaire will be completed before and after participants have the opportunity to have hands-on experience with ADS truck technology to assess a change in sentiment. The plan is to share these experiences at four “road shows” at existing trucking conferences around the country (Gallagher, 2021). Recently, TuSimple, a start-up building technology specifically designed for autonomous trucks signed their first contract with Union Pacific, to coordinate their freight shipments in Arizona, while they continue their test operations in Arizona and Texas, being ahead of most companies in this sector (Doll, 2022; Fisher, 2022).
Since 2019, a fully electric fully automated truck “T-Pod” from Einride has been driving on a public road in Sweden on behalf of logistics service provider DB Schenker. However, the approval, which was valid until the end of 2020, stipulated that the T-Pod, which weighs up to 26 tonnes, may only travel at 5 km/h, that the approval was only valid for a specific public road in an industrial area and that one employee was assigned to each vehicle as a supervisor who can intervene from a distance via joystick in the event of problems. The truck was highly technical did not have a driver’s cab. According to Einride, the e-truck offers space for 15 Euro pallets instead. Its regular maximum speed is capped at 85 km/h. A 280 kWh battery on board is supposed to guarantee a range of 200 km (Of-Allinger et al., 2020; Werwitzke, 2019).
In manageable, closed areas such as quarries or mines driverless vehicles are already being tested in practice. Volvo Trucks, for example, has six self-driving trucks in operation at a Norwegian limestone plant (Böhm, 2019).
Relevant initiatives in Austria
In Upper Austria (Gunskirchen), a driverless truck will soon also be on the road in public areas. Over the next three years, it will regularly cover the approximately 600-metre route between the logistics centre of DB Schenker Austria and the engine manufacturer BRP-Rotax. The route runs partly on factory premises and partly in public areas and is to be completed in all weathers. After completion of the test phase, the driverless electric transporter will then cover the route between DB Schenker’s logistics centre and the BRP-Rotax company, which is mainly on the public road, completely autonomously from 2023. The electric vehicle is to complete the route in all weathers, whether wind, rain or snow. For freight transport, a classic diesel truck currently travels the same route several times a day (APA, 2021; DB, 2021).
Impacts with respect to Sustainable Development Goals (SDGs)
Impact level | Indicator | Impact direction | Goal description and number | Source |
---|---|---|---|---|
Individual | ADS in heavy vehicles lead to a reduction in the total cost of ownership of trucks of between 11% and 46% | + | Sustainable economic development (8,11) | Ghandriz et al., 2020a |
Systemic | Reduction in the environmental impact | + | Environmental sustainability (7,12-13,15) | Kopelias et al., 2020 |
Systemic | ADS enable higher profitability in freight transport | + | Sustainable economic development (8,11) | Wadud et al., 2016 |
Systemic | The C-ROADS platform was established to harmonise the use of C-ITS activities across Europe | + | Partnership & collaborations (17) | European Commission, 2021 |
Open questions
- How significant will the rebound effect be that results from the improvement in freight efficiency? Should other technologies/modes of transport, therefore, be preferred?
- Who is responsible if the technology in semi-automated mode violates a speed limit or other traffic regulations?
- How should commercial CAVs best be integrated with conventional trucks?
References
- Alessandrini, A., Campagna, A., Site, P. D., Filippi, F., & Persia, L. (2015). Automated vehicles and the rethinking of mobility and cities. Transportation Research Procedia, 5, 145–160. https://doi.org/10.1016/j.trpro.2015.01.002
- Anderson, J., Kalra, N., Stanley, K., Sorensen, P., Samaras, C., & Oluwatola, O. (2016). Autonomous Vehicle Technology: A Guide for Policymakers. In Autonomous Vehicle Technology: A Guide for Policymakers. https://doi.org/10.7249/rr443-2
- APA. (2021). Selbstfahrender Lkw in OÖ unterwegs | SN.at. Salzburger Nachrichten. https://www.sn.at/wirtschaft/oesterreich/selbstfahrender-lkw-in-ooe-unterwegs-99060673
- Böhm, M. (2019, November 5). Selbstfahrende Lkws: Die Autonomen rollen an - Transport & Logistik - derStandard.at › Wirtschaft. https://www.derstandard.at/story/2000110697906/selbstfahrende-lkws-die-autonomen-rollen-an
- Boudway, I. (2021, May 5). Supply Chains Latest: Driverless Trucks a Deal Closer to Freeway. - Bloomberg. Bloomberg. https://www.bloomberg.com/news/newsletters/2021-05-05/supply-chains-latest-driverless-trucks-a-deal-closer-to-freeway
- Brown, A., Gonder, J., & Repac, B. (2014). An Analysis of Possible Energy Impacts of Automated Vehicles BT - Road Vehicle Automation (G. Meyer & S. Beiker (eds.); pp. 137–153). Springer International Publishing. https://doi.org/10.1007/978-3-319-05990-7_13
- Chan, C. Y. (2017). Advancements, prospects, and impacts of automated driving systems. International Journal of Transportation Science and Technology, 6(3), 208–216. https://doi.org/10.1016/j.ijtst.2017.07.008
- Chaudhry, A., Sha, H., Haouari, R., Quddus, M., Thomas, P., Boghani, H., Weigermars, W., Gebhard, S., Sigh, M. K., Morris, A. (2022). Evaluating the Network-Level Road Safety Impacts of Connected and Automated Vehicles in Mixed Traffic Using Traffic Microsimulation Methods. Transport Research Board. Conference: 101st Annual Meeting. Available at: https://trid.trb.org/view/1909507 [Accessed: 24 August 2022]
- DB. (2021, March 16). Autonomes Fahren: DB Schenker und BRP-Rotax starten Testbetrieb in Österreich. https://www.dbschenker.com/at-de/ueber-uns/presse/corporate-news/autonomes-fahren--db-schenker-und-brp-rotax-starten-testbetrieb-in-oesterreich-688986
- Dekhtyaruk, M. T., Shao, M., Yang, S., Kontrobayeva, Z. D., Vashchilina, E. (2021). Automated system of freight traffic optimisation in the interaction of various modes of transport. Periodicals of Engineering and Natural Sciences. 9(3). http://dx.doi.org/10.21533/pen.v9i3.2320
- Doll, S. (2022). Union Pacific becomes TuSimple’s first fully-autonomous truck customer, moving freight between rail and first/last mile without a driver. Electrek. Available at: https://electrek.co/2022/02/02/union-pacific-becomes-tusimples-first-fully-autonomous-trucking-customer-moving-freight-between-rail-and-first-last-mile-without-a-driver/ [Accessed: 24 August 2022]
- Eddy, N. (2021, May 8). Driverless trucks worth the long-term investment – Urgent Comms. https://urgentcomm.com/2021/05/10/driverless-trucks-worth-the-long-term-investment/
- European Commission. (2019). Automated road transport.
- European Commission. (2021, April 30). Connected and automated mobility | Shaping Europe’s digital future. https://digital-strategy.ec.europa.eu/en/policies/connected-and-automated-mobility
- European Commission. (2022). European Climate Infrastructure and Environment Executive Agency (CINEA). Projects: Transport and Mobility (Horizon 2020). Available at: https://cinea.ec.europa.eu/our-projects_en [Accessed: 24 August 2022]
- Fisher, J. (2022). As excitement of self-driving trucks grows, fleets will soon have products to choose from. FleetOwner: Technology. Available at: https://www.fleetowner.com/technology/article/21213982/as-excitement-of-selfdriving-trucks-grows-fleets-will-soon-have-products-to-choose-from [Accessed 24 August 2022]
- Flämig, H. (2016). Autonomous vehicles and autonomous driving in freight transport. In Autonomous Driving: Technical, Legal and Social Aspects (pp. 365–385). Springer Berlin Heidelberg. https://doi.org/10.1007/978-3-662-48847-8_18
- Gallagher, J. (2021, April 23). FMCSA automated trucks project headed to White House - FreightWaves. Freightwaves. https://www.freightwaves.com/news/fmcsa-automated-trucks-project-headed-to-white-house
- Gan, M., Qian, Q., Li, D., Ai, Y., & Liu, X. (2021). Capturing the swarm intelligence in truckers: The foundation analysis for future swarm robotics in road freight. Swarm and Evolutionary Computation, 62, 100845. https://doi.org/10.1016/j.swevo.2021.100845
- Ghandriz, T., Jacobson, B., Laine, L., & Hellgren, J. (2020a). Impact of automated driving systems on road freight transport and electrified propulsion of heavy vehicles. Transportation Research Part C: Emerging Technologies, 115, 102610. https://doi.org/10.1016/j.trc.2020.102610
- Ghandriz, T., Jacobson, B., Laine, L., & Hellgren, J. (2020b). Optimization data on total cost of ownership for conventional and battery electric heavy vehicles driven by humans and by automated driving systems. Data in Brief, 30. https://doi.org/10.1016/j.dib.2020.105566
- Harper, C. D., Hendrickson, C. T., & Samaras, C. (2016). Cost and benefit estimates of partially-automated vehicle collision avoidance technologies. Accident Analysis and Prevention, 95, 104–115. https://doi.org/10.1016/j.aap.2016.06.017
- Kalra, N., & Paddock, S. M. (2016). Driving to safety: How many miles of driving would it take to demonstrate autonomous vehicle reliability? Transportation Research Part A: Policy and Practice, 94, 182–193. https://doi.org/10.1016/j.tra.2016.09.010
- Khan, A., Harper, C. D., Hendrickson, C. T., & Samaras, C. (2019). Net-societal and net-private benefits of some existing vehicle crash avoidance technologies. Accident Analysis and Prevention, 125, 207–216. https://doi.org/10.1016/j.aap.2019.02.003
- Kopelias, P., Demiridi, E., Vogiatzis, K., Skabardonis, A., & Zafiropoulou, V. (2020). Connected & autonomous vehicles – Environmental impacts – A review. Science of the Total Environment, 712, 135237. https://doi.org/10.1016/j.scitotenv.2019.135237
- Kulikowska-Wielgus, A. (2019, March 28). Every fifth truck driver position in Europe is vacant. Soon, there could be twice as many. - Trans.INFO. Trans.Info. https://trans.info/en/every-fifth-truck-driver-position-in-europe-is-vacant-soon-there-could-be-twice-as-many-131212
- Lozzi, G., Gatta, V. Marcucci, E. (2018). European urban freight transport policies and funding: Are priorities and Horizon 2020 calls aligned. Region. 5(1), 53-71. DOI: 10.18335/region.v5i1.168
- Mersky, A. C., & Samaras, C. (2016). Fuel economy testing of autonomous vehicles. Transportation Research Part C: Emerging Technologies, 65, 31–48. https://doi.org/10.1016/j.trc.2016.01.001
- Mulholland, E., Teter, J., Cazzola, P., McDonald, Z., & Ó Gallachóir, B. P. (2018). The long haul towards decarbonising road freight – A global assessment to 2050. Applied Energy, 216, 678–693. https://doi.org/10.1016/j.apenergy.2018.01.058
- Nowakowski, C., Shladover, S. E., & Tan, H. S. (2015). Heavy Vehicle Automation: Human Factors Lessons Learned. Procedia Manufacturing, 3, 2945–2952. https://doi.org/10.1016/j.promfg.2015.07.824
- Of-Allinger, A., Knecht, J., & Conrad, B. (2020, April 30). Automomer Elektro-Lkw Einride T-Pod: Zulassung in Schweden | AUTO MOTOR UND SPORT. Auto Motr Sport. https://www.auto-motor-und-sport.de/elektroauto/automomer-elektro-lkw-einride-t-pod-strassenzulassung/
- Paddeu, D., Denby, J. (2022). Decarbonising road freight: Is truck automation and platooning an opportunity?. Clean Technologies and Environmental Policy. 24, 2021-1035. https://doi.org/10.1007/s10098-020-02020-9
- Paulsen, T. (2018, November 7). Autonomes Fahren: 5 Level zum selbstfahrenden Auto | ADAC. ADAC. https://www.adac.de/rund-ums-fahrzeug/ausstattung-technik-zubehoer/autonomes-fahren/grundlagen/autonomes-fahren-5-stufen/
- Pribyl, O., Blokpoel, R., & Matowicki, M. (2020). Addressing EU climate targets: Reducing CO2 emissions using cooperative and automated vehicles. Transportation Research Part D: Transport and Environment, 86, 102437. https://doi.org/10.1016/j.trd.2020.102437
- Sharma, S., van Lint, H., Tavasszy, L., Snelder, M. (2022). Estimating Route Choice Characteristics of Truck Drivers from Sparse Automated Vehicle Identification Data through Data Fusion and Bi-Objective Optimization. Transportation Research Record: Journal of the Transportation Research Board. https://doi.org/10.1177/03611981221095089
- Shin, S., Roh, H. S., & Hur, S. H. (2018). Technical Trends Related to Intermodal Automated Freight Transport Systems (AFTS) *. Asian Journal of Shipping and Logistics, 34(2), 161–169. https://doi.org/10.1016/j.ajsl.2018.06.013
- Tsugawa, S., Jeschke, S., & Shladovers, S. E. (2016). A review of truck platooning projects for energy savings. IEEE Transactions on Intelligent Vehicles, 1(1), 68–77. https://doi.org/10.1109/TIV.2016.2577499
- Wadud, Z. (2017). Fully automated vehicles: A cost of ownership analysis to inform early adoption. Transportation Research Part A: Policy and Practice, 101, 163–176. https://doi.org/10.1016/j.tra.2017.05.005
- Wadud, Z., MacKenzie, D., & Leiby, P. (2016). Help or hindrance? The travel, energy and carbon impacts of highly automated vehicles. Transportation Research Part A: Policy and Practice, 86, 1–18. https://doi.org/10.1016/j.tra.2015.12.001
- Werwitzke, C. (2019, May 17). Schweden: T-Pod erhält Zulassung für öffentliche Straße - electrive.net. Electrive.Net. https://www.electrive.net/2019/05/17/schweden-t-pod-erhaelt-zulassung-fuer-oeffentliche-strasse/
- Yang, S., Park, J., Abdel-Aty, M. (2022). Driver behaviour and experiences around automated freight vehicle platoons. Transport. ICE Publishing. https://doi.org/10.1680/jtran.22.00039
8.4 Automatic train operations
Updated: 29th August 2022
Synonyms
high-speed railways (HSR), automatic train control system (ATC), Grade of Automation (GoA), unattended train operation (UTO or GoA4)
Definition
Over the past decades, rail transport systems have undergone significant changes in terms of technology level, overall length, travel speed and service qualities. The management of train operations to achieve safe and efficient operation for a railway system has been a long-term concern. In traditional railway systems, this is usually achieved by (i) a timetable and a vehicle plan and (ii) real-time train operation by drivers using fixed signalling equipment. However, this partially manual work has many disadvantages for train operation due to increasing traffic demand and limited railway infrastructure. Manual driving is usually based on training and experience, which lacks rigorous calculations and systematic considerations. Therefore, it is difficult to ensure safety, quality of service (e.g. transport capacity, punctuality, accuracy of stops) and operating costs (e.g. energy consumption, infrastructure utilisation). This problem is particularly serious in urban rail systems, where passenger demand is extremely high and train departure times are very short (Yin et al., 2017).
In manual operation, drivers must keep track of the forward signals and then give appropriate train control commands. Essentially, the track logic and dispatchers only know which track circuit the train is on and prevent other trains from entering the same track circuit. Furthermore, as this process is not strictly monitored and rigorously calculated, it is usually susceptible to external factors (e.g. mental state of the driver, extreme weather that can affect driver visibility), leading to uncertainty and inefficiency (Yin et al., 2017). With the development of communication, control and computer technologies in recent decades, automatic train operation (ATO) is considered an emerging technology to replace traditional manual driving in many urban rail systems (Dong et al., 2010; Miyatake & Ko, 2010). Typically, ATO aims to improve the efficiency of railway operations by automatically making real-time decisions on optimised train acceleration, deceleration and braking commands. In the face of increasingly serious environmental and energy concerns, ATO is also widely recognised as a promising approach to reduce energy consumption and carbon emissions through optimised train control decisions while improving service quality (Yin et al., 2017).
In the railway transportation system, a distinction is made between two basic control loops: the outer control loop as the control of railway traffic and the inner control loop as the train operation itself (Rao, 2015). These two control loops are closely linked and both are important for the safe and efficient operation of a railway system (Yin et al., 2017).
Rail traffic control
Rail traffic control aims to monitor the status of traffic and infrastructure, identify deviations and conflicts, and develop a conflict-free plan for rescheduling trains to make supporting decisions for railway dispatchers to optimise the capacity and punctuality of arriving trains and avoid conflicts with other trains (Corman and Meng, 2015).
Train operations
Train operation (i.e. the inner loop) focuses on the safe and efficient train movements in each block at a microscopic level during scheduled (or rescheduled) planning by defining all the basic train control commands (i.e. accelerate, run, coast and brake).
For defining the terminology: ATO is a subsystem of the automatic train control system (ATC). ATC contains three subsystems, namely, automatic train protection (ATP), automatic train operation (ATO) and automatic train supervision (ATS) (Yin et al., 2017).
- The ATS system is responsible for monitoring train movement by performing the following tasks: Train status monitoring, automatic route selection, automatic schedule generation, automatic operation logging, statistics and reporting, and automatic system status monitoring.
- The ATP system is a fail-safe system responsible for the safe movement of each train. ATP regulates speed limits not only to maintain a safe operating distance between trains, but also to meet safety and speed requirements. Once the train exceeds the speed limit, ATP automatically applies braking (or emergency braking) to stop the train and ensure safety.
- The ATO system takes over the on-board functions of a driver to ensure smooth acceleration of the train to the running speed, speed control and precise stopping of the train at the destination platform. In normal situations, the ATO system is responsible for all control commands for traction and braking control of the train and is therefore key to the operational efficiency and profitability of train operation systems (Dong et al., 2010).
Essentially, ATO consists of computer programming and control techniques that support (or completely replace) the driver in the automatic control of train movements under the supervision of ATP and ATS. Typically, train operation is currently realised in two ways:
(1) Manual driving, supported by a driver advisory system (DAS), which can give the driver a recommended speed to help him with better driving strategies.
(2) Semi- or fully automatic mode through ATO system in light rail lines with higher level of automation, which can partially (or fully) replace manual driving. In some automated light rail lines, the ATO system first generates a recommended speed profile before the train departs, which allows the train to arrive on time at the next station. After the train has left the station, ATO’s speed controller automatically adjusts the control commands for train acceleration, coasting, running or braking through a feedback control loop. Furthermore, ATO is responsible for the automatic precise stopping of the train in the station. Other functions include the automatic reversing of trains at terminals (Yin et al., 2017).
An ATO system consists of two components (Tasler & Knollmann, 2018):
- The trackside component ATO-TS (ATO trackside) collects static and dynamic route and timetable data from existing and timetable data from existing trackside TMS (Traffic Management System) and transmits it to the ATO on-board units.
- The on-board component ATO-OB (ATO onboard) calculates the optimal the optimal driving profile at any time based on the data on infrastructure, route and timetable information, and timetable information and controls the vehicle’s traction and braking devices of the vehicle for automated driving.
According to Lang (2019), due to cost, the additional technical equipment is mainly placed inside the train when automating the railway. Tracks or stations remain largely unchanged. However, the current sensors are not designed for continuous operation in a train, for temperatures of -25 to +50 degrees, snow, rain and continuous vibrations. A factor of uncertainty for the automation of trains in regular traffic is also the acceptance of passengers. For example, the study by AutoBahn2020 shows that passengers want to have railway personnel on board, however, it does not have to be the driver.
The automation levels of train operating systems are defined by the international standard IEC 62290-1 2014. The five levels of automation range from GoA0 to GoA4. According to this standard, GoA0 and GoA1 are essentially non-automated train operation levels that require manual operation of the trains by the crew in the driver’s cab. In GoA2, acceleration and braking are automated, while the driver in the cab is responsible for the safe departure of the train and door control. GoA3 is driverless train operation, where there is no driver in the front cab of the train. Instead, there is only one member of the operating staff responsible for the safe departure of the train. Most of the existing ATO systems usually achieve GoA2 or GoA3. The highest level of train operation automation is unaccompanied train operation (Fully automated [or unattended] train operation (termed as UTO or GoA4), where there is no driver or operations staff at all and trains are operated fully automatically (Yin et al., 2017).
A wide range of automation functions are already in use on the railways today: electronic interlockings control and secure the routes, automatic train control systems ensure that train movements are secured with the greatest possible track capacity and intelligent operations control systems take over the timely setting of routes and ensure that the existing capacities are better utilised by exploiting all performance reserves and that a high level of performance and quality of rail traffic is guaranteed even in exceptional operational situations. With digitalisation, however, a further increase in performance is possible through the optimisation of complete operational processes in rail traffic according to operational and economic indicators. In the case of underground railways, fully automated rail operations have long been a reality, as their routes in closed tunnel systems make them particularly well suited for driverless operation (Tasler & Knollmann, 2018).
The expected advantages of ATO in long-distance rail transport are (Tasler & Knollmann, 2018):
- Increased line and transport capacity by reducing the intervals between trains
- Improvement of timetable stability and punctuality through uniform and plannable travel times between stations
- Energy savings through optimised driving
- Reduction of mechanical stress and wear in the drive and braking system with lower maintenance costs
- Noise reduction, especially in freight transport, through smooth and even driving smooth driving with fewer braking operations
- Increased passenger comfort due to smooth and even driving and consistent and consistent ride quality
- Increased flexibility for demand-responsive train services (with GoA3/4)
- Improvement of operating costs by increasing the effectiveness of the personnel (for GoA3/4)
Further, there is a clear lane guidance on the railways, to which the obstacle detection of fully automated systems can be limited, however, it is difficult to obtain approval in the railway sector. While an autonomously driving car can be manually controlled again immediately in case of doubt, this is not possible with a train that has no driver on board. Similarly, not all hazards can be programmed in advance. Therefore, the probability values for dangers in certain scenarios are calculated. If the system detects a person on the tracks, it estimates the probability of a collision and reacts with emergency braking, speed reduction, triggering of the signal horn etc. Emergency braking is the non-favoured means, as the braking distances of heavy trains are too long to stop in front of an obstacle. If, for example, a car crosses the tracks, in most cases it will have left the tracks long before the train reaches the crossing. The autonomous system must be able to estimate this (Lang, 2019).
Key stakeholders
- Affected: Passengers, Train operators
- Responsible: National Governments, City Governments, Private Transport Companies, Public Transport Authorities, Policymakers
Current state of art in research
A majority of ongoing research is considered to be in private hands, however, recent literature review shows some important trends in rail automation research.
Firstly, the concept of rail platooning is being developed by Schwerdfeger et al. (2021). It is to enable the coupling of several smaller trains (together with their locomotives) to form a platoon. Radio communication and sensors ensure coordination between the locomotives so that all trains in a platoon brake and accelerate at the same time (Castagnetti et al., 2016). Unlike truck platooning, where the trucks are only connected via wireless vehicle-to-vehicle communication technology, in the rail platooning concept the trains are actually physically connected. In marshalling yards, goods trains are supposed to wait for other trains to be coupled into longer train formations. However, the construction of these fast marshalling yards requires a massive investment in rail infrastructure. Germany’s largest rail operator, Deutsche Bahn, has initiated a project to evaluate whether the gains promised by train platooning are worth the huge investment. However, train platooning has the potential to significantly increase train throughput in a congested rail corridor and minimise total train kilometres if the shunting process is fully automated and takes only a few minutes. Therefore, technological developments to realise fast shunting operations are a critical success factor for the rail platooning concept (Schwerdfeger et al., 2021). For example, the Digital Automatic Coupling (DAK) is a pioneering innovation in European rail freight transport. It not only enables largely automated coupling for the first time, but also creates the conditions for the automation and digitalisation of rail freight transport in Europe and is, thus, regarded as an important step towards increasing its attractiveness and productivity (Rieder, 2020).
Secondly, the obstacle detection plays an important role in automatic train operation. To overcome the low accuracy and poor real-time performance of conventional detection methods and better detect obstacles over medium and long distances, obstacle detection in rail transport based on Deep Learning was investigated (He et al., 2021).
Although there is currently no common model or approach aimed at controlling the entire railway network, there is an emerging trend to use integrated optimisation models to simultaneously generate train control actions (e.g. recommended speed profile) and rescheduling. Furthermore, as LTE-R (Long Term Evolution for Railway) technology is emerging, enabling cross-border train-to-infrastructure and train-to-train communication, integrated rail traffic control can also benefit from the future application of this new technology and contribute to the improvement of rail transport systems (Yin et al., 2017).
Current state of art in practice
Currently, ATO is being deployed on many newly established metro mass transit lines. Almost a quarter of the world’s metro systems use at least one line in unattended train operation (UTO) mode, for example in Paris, Beijing, Dubai and Sydney (Fraszczyk & Mulley, 2017) and it has shown its great benefits in reducing manual labour, increasing the transport capacity of the infrastructure and improving the quality of service for passengers (e.g. higher punctuality and more precise stops) (Yin et al., 2017).
However, UTO operation is not yet possible in regional and long-distance traffic. Although research and facilities for the forced braking of trains and the influencing of traction units began as early as the 1930s, it was not until December 1989 that the representatives of all EU states met to decide on the creation of a uniform train control system. The planners were primarily concerned with cross-border traffic. With the uniform European Train Control System (ETCS), an internationally valid standard was to be created that other states and railway companies could then also adopt. So far, the EU Commission has mainly pushed the equipment of the international corridors, which are to be operated with ETCS throughout by the mid-2020s. In addition, new locomotives are to be generally equipped with this system, but for the time being they will also be able to run without the system with conventional signals. The achievements of the ETCS are remarkable and show the direction in which European rail traffic will go in the future. However, the introduction of the complex and technically advanced systems is associated with enormous investments (DB, 2019).
The train control system (ETCS), the train radio system (GSM-R) and parts of the uniform regulations (Technical Specifications for Interoperability (TSI)) together form the European Rail Traffic Management System (ERTMS) (ÖBB, n.d.). The ETCS enables driving without main and advance signals and increases safety at the same time. The system is comparable to an autopilot, which has been common in aviation for decades. The system monitors the train using information from the route atlas, a precise position determination and predefined command variables and can, thus, make the right decisions in good time to ensure the train’s journey even at high speeds. As in the aircraft cockpit, however, the train driver has the final say, he can drive and control the train even if the system fails or other unforeseen events occur. So although, it would technically be possible to operate the train without a driver, in the current state, it is the driver who controls the safety of the systems on the track. He still bears the responsibility for the train and its passengers or its freight. He observes the track and makes sure that there is no damage or obstacles and is the last and most important safety instance in the system. In addition, so far only a few routes are equipped with the technically demanding and price-intensive system. As soon as the train is to continue on other sections of track, the driver is also indispensable (DB, 2019).
Ongoing projects
Together with other partners, Siemens Mobility has won two innovative research projects of the German Centre for Rail Transport Research (DZSF) to investigate the safety of automated rail operations at the end of 2020. The aim is to define the necessary criteria for the approval of fully automated regional and long-distance transport. The two projects are funded with 1.7 million euros and have a duration of 30 months. The studies will focus on the highest levels of automation (GoA 3 and GoA 4), which classify fully automated trains with and without attendants. It is expected that automated regional and long-distance rail transport will shape the future of mobility. In Hamburg, Siemens Mobility is conducting a pilot project on highly automated driving with the S-Bahn, which is scheduled to start passenger operations in 2021 for the ITS World Congress. In London, Siemens Mobility has combined a system for automated train operation (ATO) with the European Train Control System (ATCS) for the first time on the ThamesLink line (Global Railway Review, 2020).
Alstom has been awarded an innovation prize for a planned test project to implement Automatic Train Operation (ATO) in the daily operation of regional passenger transport. Alstom will launch this project in 2021 together with the Regional Association “Großraum Braunschweig”, the German Aerospace Centre (DLR) and the Technical University of Berlin (TU Berlin). It will run for three years. The test trains will run between Braunschweig and Wolfsburg (Sapién, 2020). Another test by Alstom, testing obstacle detection system (ODS) took place in the Netherlands early 2022 and will be tested now in combination with ATO to pave the way for GoA4 in freight (Thibaut, 2022).
Nokia will work with Deutsche Bahn to develop 5G for ATO in 2021 on a 23-km section of Line 21 of the Hamburg S-Bahn from Berliner Tor to Bergedorf. Line 21 will initially demonstrate driverless shunting of empty trains in the Bergedorf station area based on the transmission of train control information via the 5G network (which is still being developed globally, now, in a partnership with Atos, since beginning of 2022 (Atos, 2022)). Siemens will install a similar system in Hamburg (Briginshaw, 2019).
EAST Japan Railway (JR East) has announced plans to start automatic train operation (ATO) on an initial 30km section of the Tokyo Joban commuter line between Ayase and Toride in 2021 (Cuenca, 2021). In early 2022, on Tokyo’s Yamanote loop, an automated commuter train (running through 34 stations) has been tested without passengers and showed an energy reduction of 12%. Further two months of testing will start in October and are expected to conclude in 2023 before starting improvements on train cars in the same year. EAST Japan Railway plans to implement trains in 2028 (Augusteijn, 2022).
China Railway Corporation is developing a train control system that will allow Fuxing high-speed trains to run automatically at speeds of up to 350 km per hour. The automated trains are scheduled to enter service in 2022 ahead of the opening of the Winter Olympics (China Plus, 2019). On 24th April 2022 the train was operated for the first time with its new technology verification platform for high speed EMU (electric multiple units) and reached a new world record breaking a relative crossing speed of 870km/h (single-train speed of 435km/h) on an open track section in China (Zheng, 2022).
With its smartrail 4.0, the Swiss railway SBB is only aiming for the GoA2 automation level. The ATO assistance system developed and tested by SBB is based on the European safety standard ETCS (SBB, n.d.). The train manufacturer Bombardier wants to put the first autonomous trains on the rails in long-distance transport in 2025. On closed lines, self-driving rail vehicles have already existed since the 1980s, for example in suburban and underground trains. On open tracks, however, the demands on the technology are much higher because it has to detect signals and obstacles such as fallen trees or animals without any doubt. However, development is progressing. Oz Ural (head of digital at Bombardier Transportation in Berlin) says: “Most of the technology is already there and tests are already being carried out. What is still missing for the use of autonomous trains on open tracks is the legal framework and public acceptance.” (Schwär, 2020). Nevertheless, at the end of 2020, the BAV (federal office of transport in Switzerland) gave instruction to put projects like ATO (that run under the smartrail 4.0 program) on hold. The Swiss Railway SBB did not continue further steps towards ATO. The key factor for such decision is that European system, when compared to international ATO systems, is mostly run under “mixed operations”, while other systems (e.g. Japan) use separate rails for fast and slow trains (Fischer, 2020).
The automated trains operated by the company Rio Tinto have already covered 1 million kilometres autonomously in Western Australia in 2018. The network is the world’s first autonomous heavy haul transport over long distances by rail. Rio Tinto operates about 200 locomotives on more than 1,700 kilometres of track in the Pilbara, transporting ore from 16 mines to four port terminals. Locomotives with AutoHaul software are equipped with on-board cameras that enable constant monitoring by the operations centre. All public level crossings on the network are equipped with CCTV cameras and have been upgraded to the highest safety standards (Rio Tinto Group, 2018).
Relevant initiatives in Austria
The Digital Automatic Coupling (DAK) is a pioneering innovation in European rail freight transport. It not only enables largely automated coupling for the first time, but also creates the conditions for the automation and digitalisation of rail freight transport in Europe and is, thus, regarded as an important step towards increasing its attractiveness and productivity. In February 2022, DAK was tested the first time in Austria by ÖBB (Austrian Federal Railways) (ÖBB, 2022).
Currently, about 300 km of ETCS Level 2 are in operation in Austria. In three implementation phases, the ETCS network is to be extended to 3,700 km of ETCS Level 2. From 2025, only railways that can operate with this standard will be allowed to use it. The current national program runs until 2026 including all main routes, followed by more detailed programs running until 2030 and 2038.
From 2025, driverless metro trains are to run on the new U5 line in Vienna. In 2022 the U2/U5 station Volkstheater was the first to be equipped with automatic platform doors, which is a mandatory safety measure to run a driverless train in Vienna (Krassay, 2022).
In the climate fund project AutoBahn2020, researchers from the FH Upper Austria have taken on the automation of trains. On the local railway between Vorchdorf and Gmunden they tested a train upgraded with technology in driverless operation. Possible applications for autonomously driving trains are seen primarily in regional railways. With additionally deployed driverless trains, the frequency of travel could be increased without increasing personnel costs at the same time. On the other hand, routes with more frequent traffic, such as between Vienna and Salzburg, are not suitable: “Here, the personnel costs only play a subordinate role because of the high utilisation. However, routes that have been discontinued due to a lack of economic efficiency could be revived by means of autonomous trains.”
Impacts with respect to Sustainable Development Goals (SDGs)
Impact level | Indicator | Impact direction | Goal description and number | Source |
---|---|---|---|---|
Individual | Better punctuality and smoother acceleration and braking trains | + | Health & Wellbeing (3) | Yin et al., 2017 |
Systemic | Noise reduction and increased safety due to several automated systems | + | Health & Wellbeing (3) | Tasler & Knollmann, 2018 |
Systemic | Reduced energy consumption | + | Environmental sustainability (7,12,13,15) | Tasler & Knollmann, 2018; Yin et al., 2017 |
Systemic | Parallel efforts in different countries on the development of ATO | + | Innovation & Infrastructure (9) | China Plus, 2019; Cuenca, 2021; Briginshaw, 2019 |
Systemic | Many collaboration and joint projects emerge | + | Partnership & collaborations (17) | Sapien, 2020; Briginshaw, 2019 |
Open questions
- How to deal with the social impact of train drivers being able to lose their jobs?
- How to increase social acceptance of top level automated trains in Europe?
References
- AtoS (2022). Atos and Noia join forces to enhance businesses’ ditital applications with full private 4/5G networks services. Available at: https://atos.net/en/2022/press-release_2022_02_24/atos-and-nokia-join-forces-to-enhance-businesses-digital-applications-with-full-private-4-5g-networks-services [Accessed: 27th August 2022]
- Augusteijn, N. (2022). East Japan Railway plans trial runs with automated commuter train. Available at: https://www.railtech.com/rolling-stock/2022/05/12/east-japan-railway-plans-trial-runs-with-automated-commuter-train/?gdpr=accept [Accessed: 27th August 2022]
- Briginshaw, D. (2019, December 12). DB appoints Nokia to develop 5G network for automatic operation | International Railway Journal. https://www.railjournal.com/infrastructure/db-appoints-nokia-to-develop-5g-network-for-automatic-operation/
- Castagnetti, F., Toubol, A., & Rizzi, G. (2016). C4R Project Increases Rail Capacity without Laying Down New Tracks. Transportation Research Procedia, 14, 672–678. https://doi.org/10.1016/j.trpro.2016.05.329
- China Plus. (2019, January 4). China developing world’s first 350 km/h automated bullet trains - China Plus. http://chinaplus.cri.cn/news/china/9/20190103/231172.html
- Cuenca, O. (2021, February 22). JR East to introduce ATO on the Joban Line next month | International Railway Journal. https://www.railjournal.com/regions/asia/jr-east-to-introduce-ato-on-the-joban-line-next-month/
- DB. (2019, March 19). ETCS: Das Europäische Zugsicherungssystem | DB Inside Bahn. https://inside.bahn.de/etcs-europaeisches-zugsicherheitssystem/
- Dong, H., Ning, B., Cai, B., & Hou, Z. (2010). Automatic Train Control System Development and Simulation for High-Speed Railways. IEEE Circuits and Systems Magazine, 10(2), 6–18. https://doi.org/10.1109/MCAS.2010.936782
- Fischer, M. (2020). Smartrail 4.0: Utopie und Realität – BAV pfeift Bahnen zurück. SEV. Available at: https://sev-online.ch/de/aktuell/kontakt.sev/2020/utopie-und-realitt-202019-35343/ [Accessed: 28 August 2022]
- Fraszczyk, A., & Mulley, C. (2017). Public Perception of and Attitude to Driverless Train: A Case Study of Sydney, Australia. Urban Rail Transit, 3(2), 100–111. https://doi.org/10.1007/s40864-017-0052-6
- Global Railway Review. (2020, November 24). Siemens Mobility and partners to study automated rail operations. https://www.globalrailwayreview.com/news/114422/siemens-mobility-partners-automated-rail-operations/
- He, D., Zou, Z., Chen, Y., Liu, B., Yao, X., & Shan, S. (2021). Obstacle detection of rail transit based on deep learning. Measurement: Journal of the International Measurement Confederation, 176, 109241. https://doi.org/10.1016/j.measurement.2021.109241
- Krassay, B. (2022). Wien rüstet für die fahrerlose U-Bahn. Die Presse. Available at: https://www.diepresse.com/6176532/wien-ruestet-fuer-die-fahrerlose-u-bahn [Accessed: 28th August 2022]
- Miyatake, M., & Ko, H. (2010). Optimization of train speed profile for minimum energy consumption. IEEJ Transactions on Electrical and Electronic Engineering, 5(3), 263–269. https://doi.org/10.1002/tee.20528
- ÖBB. (n.d.). ETCS Zugbeeinflussung - ÖBB-Infrastruktur AG. Available at: https://infrastruktur.oebb.at/de/geschaeftspartner/schienennetz/dokumente-und-daten/etcs-zugbeeinflussung [Accessed: 14 May 2021]
- ÖBB (2022). Die Digitale Automatische Kupplung. Available at: https://www.unsereoebb.at/de/dak-22 [Accessed: 28 August 2022]
- ÖBB (2022). Rückblick: Der DAC4EU Demonstratorzug in Österreich. Available at: https://blog.railcargo.com/de/artikel/Der-DAC4EU-Demonstratorzug-in-Oesterreich [Accessed: 28th August 2022]
- Rao, X. (2015). Holistic rail network operation by integration of train automation and traffic management. PhD Thesis, ETH Zurich, 22706.
- Rieder, B. (2020). ÖBB: Fokus auf Digitaler Automatischer Kupplung (DAK) im Güterverkehr. Available at: https://presse.oebb.at/de/presseinformationen/20200908-oebb-fokus-auf-digitaler-automatischer-kupplung-im-gueterverkehr [Accessed: 20th October 2021]
- Rio Tinto Group. (2018, December 28). World-first autonomous trains deployed at Rio Tinto’s iron ore operations. https://www.riotinto.com/news/releases/World-first-autonomous-trains-deployed
- Sapién, J. C. (2020, May 28). Alstom Wins Prize for World-First: ATO Testing for Regional Trains | Railway-News. https://railway-news.com/alstom-wins-german-innovation-prize-ato/
- SBB. (n.d.). Automatic Train Operation (ATO): die innovative Automatisierung | SBB. Available at: https://bahninfrastruktur.sbb.ch/de/digitale-bahn/ato.html [Accessed: 5th May 2021]
- Schwär, H. (2020, July 24). Autonome Züge ab 2025: Wie sich Bombardier zukunftsfit machen will - Business Insider. https://www.businessinsider.de/wirtschaft/mobility/autonome-zuege-ab-2025-und-schnelles-internet-im-abteil-wie-dieser-30-jaehrige-den-zughersteller-bombardier-fit-fuer-die-zukunft-machen-will/
- Schwerdfeger, S., Otto, A., & Boysen, N. (2021). Rail platooning: Scheduling trains along a rail corridor with rapid-shunting facilities. European Journal of Operational Research. https://doi.org/10.1016/j.ejor.2021.02.019
- Tasler, G., & Knollmann, V. (2018). Einführung des hochautomatisierten Fahrens – auf dem Weg zum vollautomatischen Bahnbetrieb. Signal + Draht, 110(6), 6–14.
- Thibaut, S. (2022). Alstom takes another step towards Autonomous Train Operation in the Netherlands. Alstom. Available at: https://www.alstom.com/press-releases-news/2022/4/alstom-takes-another-step-towards-autonomous-train-operation-netherlands [Accessed 27th August 2022]
- Yin, J., Tang, T., Yang, L., Xun, J., Huang, Y., & Gao, Z. (2017). Research and development of automatic train operation for railway transportation systems: A survey. In Transportation Research Part C: Emerging Technologies (Vol. 85, pp. 548–572). Elsevier Ltd. https://doi.org/10.1016/j.trc.2017.09.009
- Zhen, R. (2022). Running at 435km/h, China’s New Fuxing High-speed Train Sets World Record. I Chong Qing. Available at: https://www.ichongqing.info/2022/04/27/run-at-435km-h-chinas-new-fuxing-high-speed-train-sets-world-record/ [Accessed: 27th August 2022]